Experts Discuss the Foundations and Future of AI at CAIT Research Showcase
A diverse group of researchers discussed recent findings, ethics, and social consequences of AI at the recent Columbia Center of Artificial Intelligence Technology (CAIT) research showcase
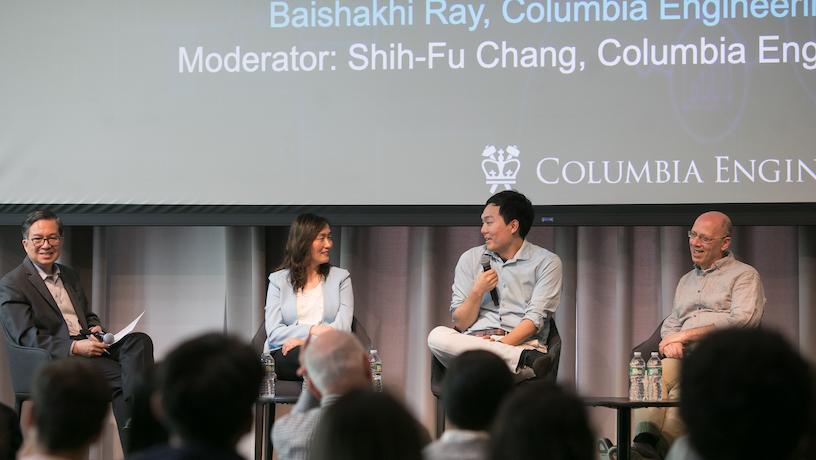
Credit: Timothy Lee
An interdisciplinary group of experts in artificial intelligence (AI) gathered May 3 at Columbia University to share their latest research findings and discuss how the rapidly advancing field will likely affect business, academia, and society. The annual Columbia Center of Artificial Intelligence Technology (CAIT) Research Showcase featured an expert panel and lightning talks given by faculty recipients of CAIT Research Awards and PhD fellowships.
The day’s main event was a panel discussion on large language models (LLMs) featuring experts from Columbia Engineering, across the University, and from CAIT sponsor, Amazon. Shih-Fu Chang, dean of Columbia Engineering and director of CAIT, moderated the discussion held at Pulitzer Hall.
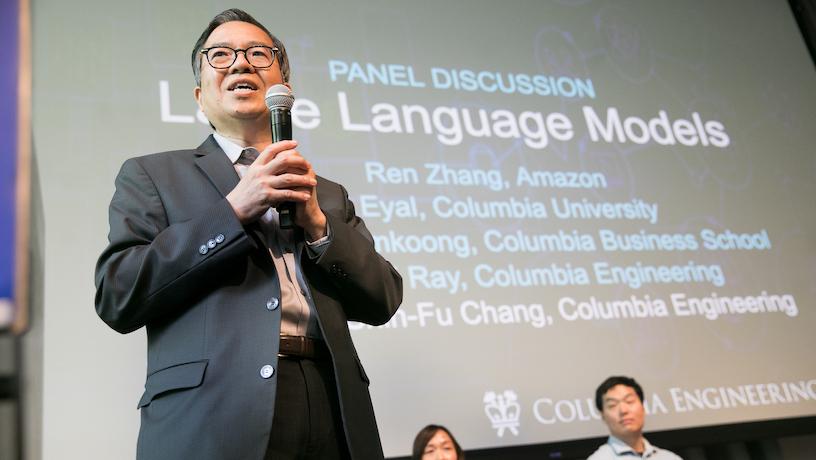
Credit: Timothy Lee
The panel explored LLMs like ChatGPT, which has garnered a lot of attention since its widespread release last fall. Said Dean Chang, “Some of you probably already have tested the potential of these systems — and seen the sometimes funny or surprising outputs of the large language models.”
He added, “Our faculty in Columbia Engineering now number over 250, and more than 50 faculty members are conducting research involving artificial intelligence in some way.”
In a lively discussion, the interdisciplinary group of panelists tackled topics ranging from practical uses for LLMs to debates around the significance of the “sparks” of artificial general intelligence some say these systems have begun to display. The biggest themes of the conference were centered on trust and ethics.
The trust factor
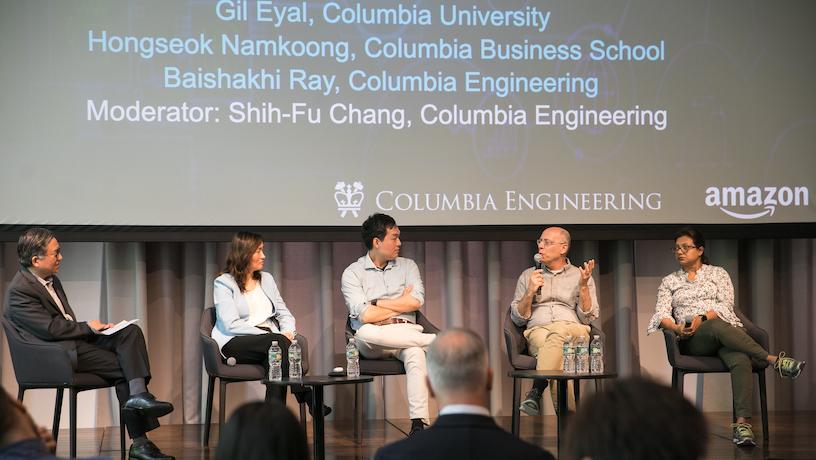
Credit: Timothy Lee
Ren Zhang, director of data science at Amazon, said “the huge leap in performance” that GPT4 represents stands to “unlock new pathways to innovation” — while bringing a new set of concerns.
“How do we find a way to guardrail the trustworthiness of AI?” she asked. “We’ve all observed the hallucinations in the demonstration of OpenAI’s cutting-edge LLM, GPT-4. I’m not sure how likely it is that they can be corrected. Maybe there will always need to be a human in the loop.”
Baishakhi Ray, associate professor of computer science at Columbia Engineering, described some technical approaches that are making modes that generate code more reliable. Her research group has integrated LLMs with the graph structure of code to design models — similar to Amazon’s CodeWhisperer, which is a program to support software engineers — that are far more robust than standard LLMs.
“If models are a little bit aware of code properties, they can be much better,” she said.
While recent advancements in AI have brought a wave of new concerns surrounding trust and ethics, panelist Gil Eyal, a professor of sociology at Columbia and director of The Trust Collaboratory, explained a practical reason for focusing on trustworthiness: People won’t use systems they don’t trust.
“The thing about trust is that it doesn't work like statistics. It doesn't work if it's 70% okay. You’re not even going to trust something that’s 95% okay,” he said.
The panelists agreed that building systems worthy of trust is essential to building and maintaining trust with users and citizens who are affected by AI. In many cases, that will require keeping humans in the loop.
Lightning Talks: AI's Foundational Technologies, Applications, and Social Impact
Over the course of the half-day conference, several CAIT PhD fellows and affiliated faculty also gave lightning talks on topics spanning many aspects of AI, from fundamental technologies to new applications.
Will Ma, an assistant professor of decision, risk, and operations at Columbia Business School, kicked off the session with a presentation describing a model that would allow large retailers to optimize their inventory decisions. The model incorporates real-world factors such as how inventory levels affect customer choices, which are ignored by today’s state-of-the-art approaches.
Matei Ciocarlie, associate professor of mechanical engineering at Columbia Engineering, presented his team’s work on deep reinforcement learning for automated systems that rely on human input when their confidence falls below a certain threshold. Along with their collaborators at Amazon, Ciocarlie’s group is developing foundational human-in-the-loop AI technologies that could be applied to automated forklifts that seek input from human teleoperators when needed.
PhD student Haoxian Chen, in the Department of Industrial Engineering and Operations Research (IEOR) at Columbia Engineering, was the first CAIT PhD fellow to present at the showcase. In a talk titled, “Scalable Black-Box Optimization via a Pseudo-Bayesian Framework,” Chen described his work with associate professor Harry Lam that improves on state-of-the-art approaches for solving some of the hardest problems in optimization.
CAIT fellow Melanie Subbiah, a computer science PhD student working with Professor Kathleen McKeown on natural language processing, described her work characterizing the abilities of large language models to summarize long narratives. While these models are able to summarize simple text, such as a news article, Subbiah found that even the most advanced LLMs are incapable of summarizing longer, more complex narratives, such as short stories or novels.
Julia Hirschberg, professor of computer science at Columbia Engineering, kicked off the second round of lightning talks with a presentation on the fundamental work she and her team are doing to understand empathy in spoken language. The researchers are using videos in English and Mandarin to build a database that will enable them to understand the speech features that correspond with empathetic content. One ultimate goal of this project is to help Amazon’s Alexa speak in a more empathetic way.
Eric Balkanski, an assistant professor in IEOR at Columbia Engineering, presented his work developing optimization techniques that will lead to much faster algorithms for a wide range of problems in machine learning. The parallel submodular techniques he described combine the benefits of parallel and distributed frameworks and have potential applications that range from computer vision to modeling the spread of infectious diseases.
CAIT fellow Tuhin Chakrabarty, a PhD candidate in computer science at Columbia Engineering, capped off the lightning talks describing his team’s novel approach of using LLMs and diffusion-based text-to-image models to create images to represent visual metaphors. The researchers developed a chain-of-thought prompting technique that enabled the text-to-image diffusion models to depict imagery based on figurative language far more effectively than previous methods.
Remote Talks
Several researchers who couldn’t attend the conference in person supplied recordings of their lightning talks. Learn more about projects ranging from large-scale edge computing to financial applications of AI.
Watch the Remote Talk | Neural Causal Models with Abstractions | Kevin Xia
Watch the Remote Talk | Scalable Computation of Causal Bounds | Madhumitha Sridharan
Watch the Remote Talk | Single-Leg Revenue Management with Advice | Rachitesh Kumar